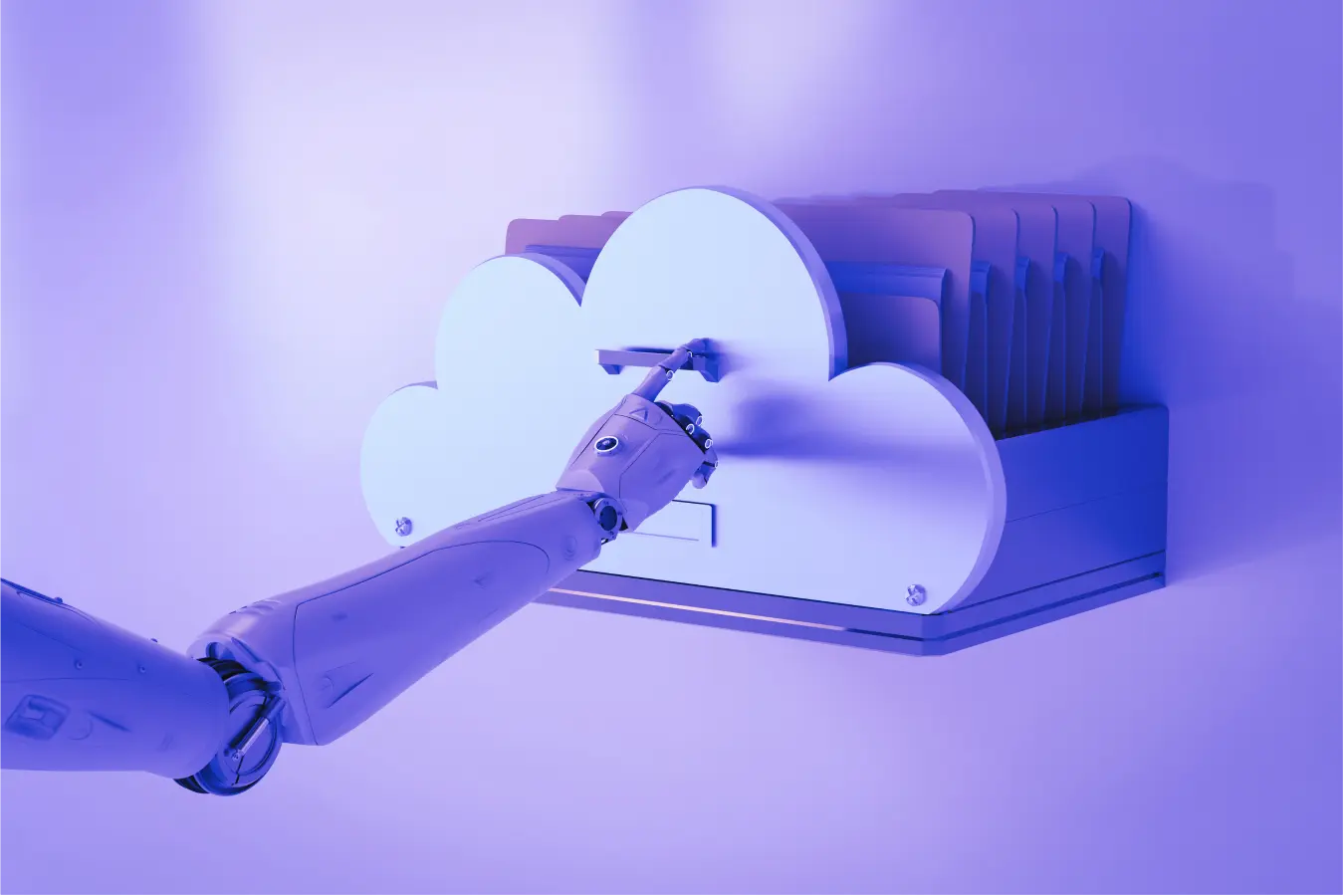
Introduction
AI infrastructure, which serves as the backbone of modern technological advancements, has significantly evolved from traditional on-premises configurations to include cutting-edge solutions such as cloud computing and edge computing. However, as organizations increasingly embrace AI, they face a decision: should they move their workloads to edge, cloud, or maintain them on-premises?
Before migrating workloads or deploying any applications, it’s essential to understand the strengths and nuances of each environment—be it cloud, edge, or on-premises. Factors such as scalability, latency, security, and cost-effectiveness are crucial and can vary significantly between these options.
To help you gain clarity on which platform might be the best fit, this article offers a comparative analysis of cloud, edge, and on-premises environments. The aim is to provide insights that can guide you in determining the optimal choice for deploying your AI initiatives.
Understanding Edge AI, AI Cloud and On-Premises AI Infrastructure
1. Edge AI Infrastructure
Edge AI combines edge computing and artificial intelligence by running machine learning models locally on devices such as computers, edge servers, and IoT devices.
Unlike traditional AI, these devices can operate without an Internet connection and make decisions independently. They not only collect data but also process and act on it in real-time, enhancing efficiency and responsiveness.
For example, virtual assistants like Google Assistant, Siri, and Alexa process user commands locally, interact with cloud APIs, and store learned data on the device, allowing for faster and more personalized interactions.
Gartner forecasts that by 2025, over 55% of all data analysis conducted by deep neural networks will take place directly at the source within an edge system. It’s crucial for organizations to recognize the applications, along with the AI training and inferencing processes, that need to be transitioned to edge environments in proximity to IoT endpoints.
2. AI Cloud
AI Cloud services, also known as AI as a Service (AIaaS), are cloud platforms offering AI resources to individuals and businesses. This technology, which combines cloud computing and artificial intelligence, transforms daily operations by integrating AI tools, algorithms, and cloud services.
This integration allows enterprises to leverage AI capabilities such as machine learning, natural language processing, and computer vision, providing a competitive edge. AI Cloud’s ability to quickly process large datasets benefits data-centric industries like e-commerce, banking, and healthcare by uncovering patterns and insights, enabling data-driven decisions.
AIaaS makes AI more accessible, scalable, and affordable. Featuring GPUs and TPUs for deep learning training, it eliminates the need for specialized hardware, democratizing access to advanced AI capabilities and revolutionizing the technology field.
The best cloud for AI varies based on project requirements, infrastructure, and budget. Top providers include:
- Microsoft: Azure OpenAI is a platform based in the cloud that empowers developers and data scientists to construct and launch AI models with speed and ease. It provides users with a broad spectrum of AI tools and technologies for crafting smart applications, encompassing areas like computer vision, and deep learning
- AWS: Offers a range of cloud services and AI technologies, including Amazon SageMaker for machine learning and Amazon Rekognition for image/video analysis.
3. On-prem AI Infrastructure
On-prem AI involves deploying and managing artificial intelligence (AI) infrastructure and applications locally within an organization’s premises or data centers. This includes handling hardware and software resources necessary for tasks like data storage, processing, and application execution, rather than relying on external cloud platforms or edge computing solutions.
Building and scaling AI projects necessitates substantial computing power. For organizations seeking greater computational capabilities, this represents a significant upfront investment even before starting. Moreover, on-premises infrastructure entails ongoing operational costs post-deployment. Unlike cloud solutions, on-prem deployments have limited access to pre-trained models and ready-made AI services that enterprises can readily leverage.
On-Premises vs. AI Cloud vs. Edge AI: Comparison Analysis
Now that you have a grasp of the evolution of AI infrastructure and its significance in the technology landscape, let’s analyze the three types of infrastructure to identify which one aligns best with your organization’s needs.
Aspect | AI Cloud | Edge AI | On-prem AI |
Location of Processing | Remote data centers managed by cloud providers. | Near the data source (e.g., devices, sensors). | Local data centers or on-premises servers. |
Scalability | Highly scalable, resources can be easily scaled up or down. | Limited scalability based on local resources. | Scalability depends on on-premises hardware and capacity. |
Latency | Higher latency due to data transmission to/from cloud. | Low latency, data processed closer to the source. | Low latency, data processed locally. |
Cost | Pay-as-you-go model, initial setup costs may vary. | Lower data transfer costs, but higher initial setup costs. | Upfront investment in hardware, ongoing operational costs. |
Accessibility | Globally accessible from anywhere. | Limited to local area network or specific devices. | Accessible within the organization’s premises. |
Managed Services | Extensive managed AI services and tools available. | Limited managed services, typically DIY approach. | Full control over AI environment and configurations. |
Privacy and Security | Potential concerns over data privacy and security but depends on cloud providers. | Enhanced privacy by keeping data local. | Enhanced security through physical control of infrastructure. |
Compliance | Compliance with data residency regulations. | Easier compliance with local data regulations. | Easier compliance with industry-specific regulations. |
Performance | Suitable for complex AI tasks requiring substantial resources. | High performance for real-time applications. | Customizable for specific performance needs. |
Benefits of Cloud and Edge AI
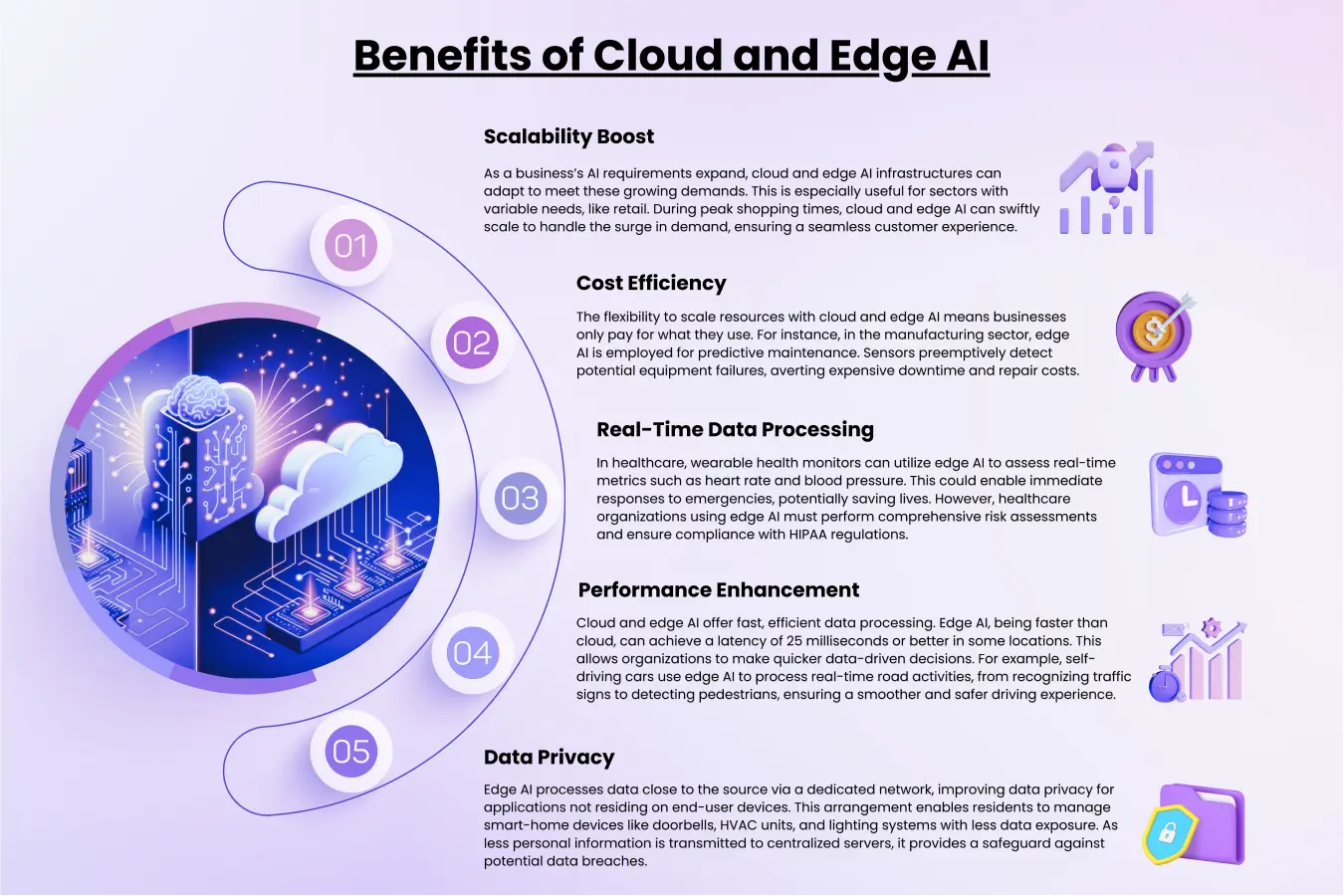
When to opt for On-Premises AI?
Real World Case Studies
- Canonical company known primarily for its development and support of the Ubuntu operating system (OS) implemented an on-premises AI infrastructure to predict S&P 500 Index trends, driven by stringent security requirements, cost considerations related to data gravity, and the need for efficient data handling in financial markets.
- This approach was chosen over public cloud solutions due to the imperative of maintaining compliance and avoiding the complexities and expenses associated with transferring large datasets off-site for AI model training.
- Their solution utilizes Elasticsearch for storing raw financial data, Kubeflow Pipelines for managing end-to-end AI workflows, including TensorFlow model training, and Ceph for distributed storage of trained models.
- TensorFlow Serving and Apache Kafka facilitate real-time model deployment and communication. Managed through Juju on Kubernetes, this setup optimizes the use of local CPU/GPU resources, ensuring robust security, cost efficiency, and high-performance AI operations tailored specifically for the demands of financial sectors.
- Schneider Electric: Schneider Electric implements Microsoft Azure IoT Edge for edge AI, optimizing energy management and predictive maintenance in smart buildings and industrial facilities through local data processing.
- Schneider-Electric is enhancing the quality of life for millions of people in Nigeria by delivering dependable, environmentally friendly, and cost-effective energy solutions powered by Azure IoT.
- Walmart: In 2024, the company aims to enhance the online shopping experience by integrating generative AI into its search feature. This improvement aims to provide customers with a more user-friendly and intuitive browsing experience.
- By leveraging Walmart’s proprietary data and technology, alongside large language models such as those accessible through Microsoft Azure OpenAI Service, as well as retail-specific models developed internally, the updated design offers personalized recommendations tailored to each shopper’s preferences.
Conclusion
Choosing the right AI infrastructure depends on your organization’s specific needs and strategic goals.
Cloud AI is ideal for scalability, cost-efficiency, and global accessibility, making it suitable for dynamic and rapidly evolving AI projects.
Edge AI is perfect for real-time applications, offering low latency, bandwidth efficiency, and enhanced privacy and security.
On-premises AI provides control, customization, and compliance, making it the best choice for regulated industries and high-performance requirements.
To conclude, a hybrid approach that combines the strengths of cloud, edge, and on-premises environments can offer the most robust solution, leveraging the unique benefits of each to optimize AI deployment models edge and performance. As AI technology continues to advance, understanding these infrastructure options will be key to unlocking its full potential for your organization.
If you’re looking to advance your AI projects, Aptly’s AI Infrastructure Readiness services could be the ideal solution. We utilize our expertise with state-of-the-art hardware, including advanced technologies such as TPUs and GPUs, across different settings: on-premises, in the cloud, and within data centers.